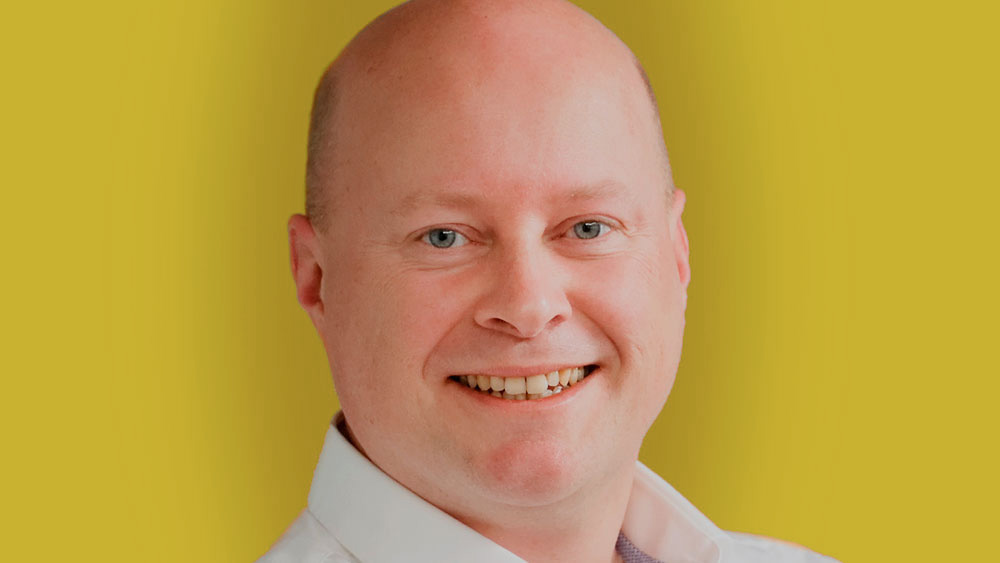
No model, no glory
Everybody wants AI, but few are ready to pay the price. After five years of providing AI engineering services in the high-tech industry, this is my conclusion. I know it’s a bold statement, but give me a moment to complain – I’m Dutch, and we have a tradition of speaking our minds frankly.
A decade ago, the mantra was “Data is the new gold.” This has shifted to “No model, no glory.” In the realm of intelligent systems, AI models have become indispensable. They crucially enhance system performance once the mechanics and electronics are fine-tuned. Key performance questions like “Do we detect all objects?”, “How many objects are wrongly classified?” and “What’s the spatial accuracy of our detection?” are now central in advancing AI technology across sectors like agriculture and manufacturing. A colleague once suggested we adopt an AI-first approach in our engineering projects to underline its importance from a systems perspective and promote its broader adoption in our solutions.
There’s a prevailing misconception that AI is straightforward and nearly cost-free, largely fueled by Big Tech companies releasing open-source AI tools and models. These offerings, especially recently in the realm of large language models, make sophisticated AI systems seem readily available without significant expense. Additionally, the way AI breakthroughs are often portrayed in the media can make the development process appear deceptively simple. Seeing highly polished AI systems in action reinforces the illusion of ease, but in reality, these systems require years of specialized engineering, extensive research and significant financial investment.
Consider, for instance, the development of an AI model designed for a berry-picking robot. Initially, data collection requires capturing about 10,000 images. An engineer traveling and processing data can produce approximately 250 images per day at a rate of 50 euros per hour, amounting to 16,000 euros over 40 days. The annotation process involves tagging an average of 100 berries per image at 0.10 euros per berry, totaling 100,000 euros. Furthermore, a typical object detection framework might incur a yearly license fee of 60,000 euros. Training the model requires GPU resources costing 4,000 euros for 100 training jobs at 10 hours each. An experienced AI engineer dedicated to the project for six months can cost about 60,000 euros, with an additional 20,000 euros for optimizing the model for embedded deployment.
Altogether, the initial investment required to operationalize a standard model, like an object detection model, can reach 200,000 euros. However, when the development involves more complex algorithms, such as those needed to create a full plant model or to estimate 3D areas or volumes, the costs and time commitments can easily quadruple. This stark increase underscores the significant resources required as project complexity increases.
However, the expenses don’t end once the model is deployed. Continuous monitoring and retraining are critical as the model must adapt to new data and conditions to maintain its accuracy and efficiency. This ongoing maintenance can be as costly as the initial development, demanding significant funds, time and expertise.
Despite the significant costs, the value these models add is undeniable. They’re transforming industries by increasing the accuracy and efficiency of manufacturing production lines, optimizing inventory management in retail and enhancing precision in semiconductor fabrication processes. In agriculture, AI significantly enhances yield predictions and automates various laborious tasks, providing a substantial return on investment that justifies the initial financial outlay. However, the challenges in agriculture are unique; the limited duration of growing seasons restricts rapid data collection. The unstructured and unpredictable nature of agricultural environments often requires a complete dataset before an AI-enabled product can pass proof-of-concept evaluations and gain market acceptance.
Every AI project demands a significant investment, encompassing not only financial resources but also considerable time and expertise. Drawing on my extensive experience in this field, I advise looking beyond the initial costs to recognize the long-term benefits and competitive advantages these technologies bring. To reduce development costs, consider partnering with experienced AI engineering firms that provide partially completed solutions, helping you avoid costly learning mistakes.
In an era where every sector seeks to leverage technology to its fullest, understanding and investing in AI isn’t merely an option; it’s a necessity. Let’s adjust our expectations and start planning for the true costs of AI, ensuring we're all ready to pay the price for the tremendous benefits it brings.